芬兰人工智能中心研发隐私计算新算法,准确测量隐私泄露
发布时间: 2021-05-11
一种隐私计算新的算法已经在谷歌发布的专用开源库和芬兰人工智能中心的开源库中得到了应用。
赫尔辛基大学和阿尔托大学的芬兰人工智能中心(FCAI)研究人员已经非常准确地计算出,当数据主体的数据被用来训练一个保护隐私的机器学习模型(如神经网络)时,他们还能保留多少隐私。
该新算法基于一个名为 "差分隐私 "的隐私框架,该框架在2020年被《麻省理工科技评论》列为将改变我们生活方式的技术候选名单。
赫尔辛基大学副教授Antti Honkela说:"差分隐私正在被用来保证谷歌和苹果公司开发的使用敏感用户数据的人工智能系统不泄露其敏感数据,以及保证美国2020年人口普查数据的隐私性"。新的算法允许在这类分析中对隐私的保护程度进行更准确的估计。
差异性隐私算法通过随机扰动计算来保护隐私。在非常复杂的算法环境中,FCAI的研究人员首次成功地准确量化了这些扰动对隐私的保护。"扰动越大,隐私要求通常就越强,但估计一个特定算法的真正隐私程度可能很困难。对于如神经网络训练这样复杂的算法,估计精确的隐私丧失会尤其困难,这可能需要动用一项叫做隐私会计的新技术",赫尔辛基大学的博士后研究员Antti Koskela说。事实上,FCAI的研究人员已经开发了一种新的隐私会计,可以提供几乎完美的准确性。
"新算法能够为相同的计算证明出更明确的隐私损失。反之,人们可以减少随机扰动的大小,在同等隐私保障下获得比以前更准确的结果",Koskela说。例如,这些结果将使我们能够以精准了解每个人隐私弱点的方式来训练机器学习模型。这将对使机器学习和人工智能的可靠性产生巨大影响。
新算法基本上提供了在差分隐私的框架下可获得的最佳保留隐私的措施。最近的研究表明,新算法可以准确衡量一家公司可以从公开的数据中获得多少私人信息。此外,使用新的、更准确的隐私界限衡量,也可以更准确地比较不同的保护隐私的机器学习算法的优劣。
这项成果已在2021年4月的国际人工智能和统计会议(AISTATS)上发表。
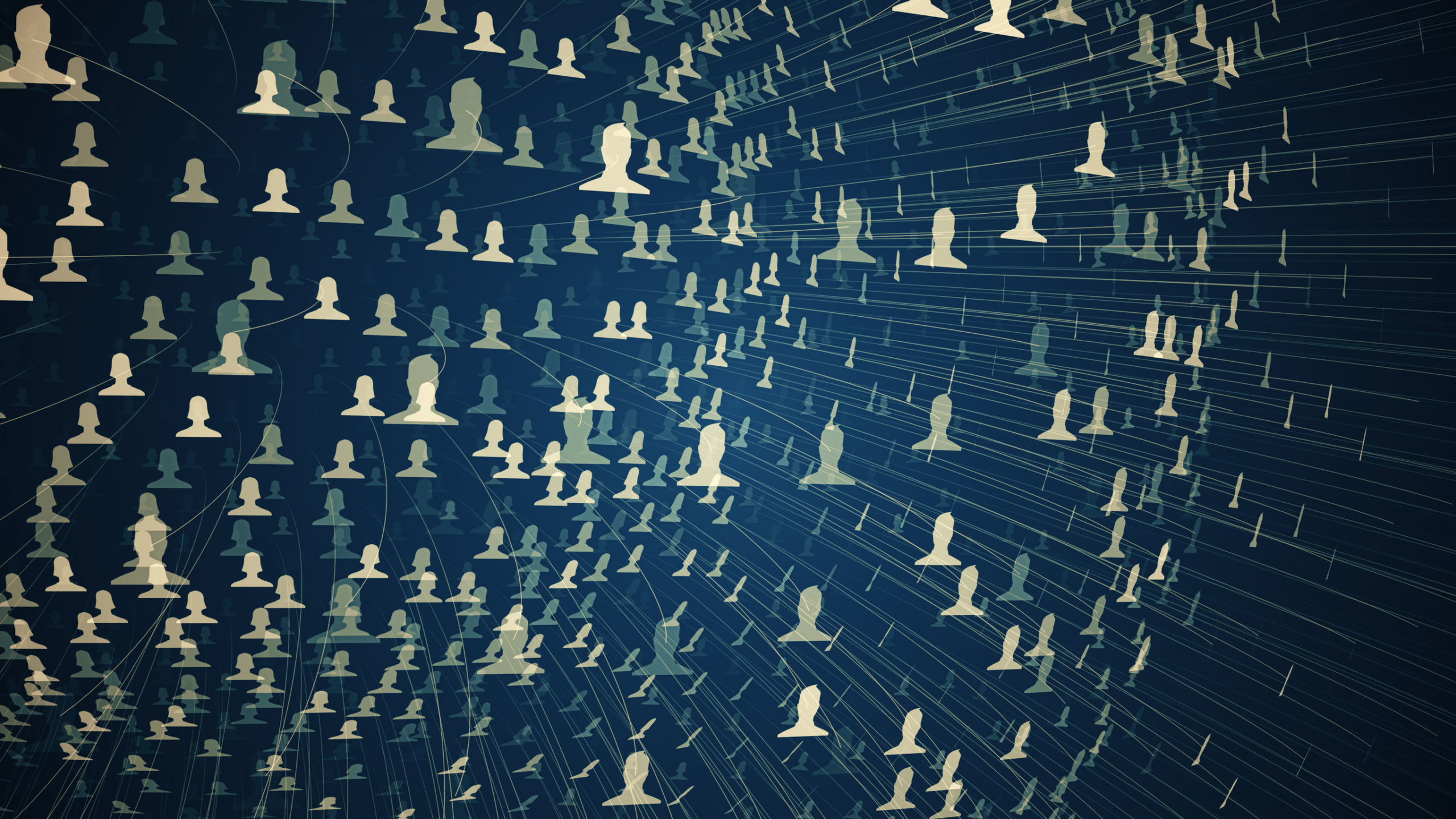
原文:
The new algorithm is already being taken into use in a dedicated open source library released by Google and to an open source library developed by FCAI researchers.
Finnish Center for Artificial Intelligence FCAI researchers at the University of Helsinki and Aalto University have figured out very accurately how much privacy data subjects retain when their data are used to train a privacy-preserving machine learning model such as a neural network.
The new algorithm is based on a privacy framework called differential privacy which was shortlisted by MIT Technology Review in 2020 as a technology that will change the way we live.
“Differential privacy is used, among others, to guarantee that AI systems developed by Google and Apple using sensitive user data cannot reveal that sensitive data, as well as to guarantee the privacy of data released by US Census 2020”, says Antti Honkela, Associate professor at the University of Helsinki.
The new algorithm allows a more accurate estimation of the retained privacy in these and many other kinds of analyses.
Differentially private algorithms randomly perturb computations to preserve privacy. FCAI researchers have succeeded, for the first time, to accurately quantify the protection of privacy that these perturbations provide even in very complex algorithms.
“The larger the perturbation, the stronger the privacy claims typically become, but estimating how private a particular algorithm really is can be difficult. Estimating the precise privacy loss is especially difficult for complex algorithms such as neural network training, and this requires using a so-called privacy accountant”, says Antti Koskela, Postdoctoral researcher at the University of Helsinki.
FCAI researchers have developed a new privacy accountant that provides almost perfect accuracy. The method comes with provable upper and lower bounds on the true privacy loss.
“The new algorithm enables the proving of stronger privacy bounds for the same computation. Conversely, one can reduce the magnitude of the random perturbations to obtain more accurate results under equal privacy guarantees than before”, says Koskela.
The results will enable, for example, training machine learning models in a way that each individual’s vulnerability to a privacy breach will be known precisely. This will have a huge impact on making machine learning and AI more trustworthy.
The new algorithm provides essentially the best obtainable measure of the retained privacy under the differential privacy formalism. Recent research suggests this is an accurate measure of how much private information a very powerful adversary could obtain from published results. More research will be needed to extend these possibly pessimistic worst-case estimates to different and more realistic scenarios. Moreover, using the new, more accurate privacy bounds, different privacy-preserving machine learning algorithms can be compared more accurately because the accuracy of privacy bounds will no longer impact the comparisons.
The work will be published at the International Conference on Artificial Intelligence and Statistics (AISTATS) in April 2021.
原文作者:Antti Koskela
本文转载自:Helsinki Institue for Information Technology www.hiit.fi
原文地址:https://www.hiit.fi/new-algorithm-reveals-how-private-privacy-preserving-computations-really-are/
-