Data Governance: Simple and Practical
发布时间: 2023-04-12
In 2022, the global data governance market grew to $3.2 billion, a figure that’s dwarfed by the significant cost to operate data governance programs. In spite of the spend, many programs fail to generate high business value because they lack practicality and simplicity: the art of maximizing the amount of work not done.
Where data governance programs often get off track is right before they get on, by defining the charter and roadmap too broadly. The key question is which elements of a data governance program should you chase out of the gate: ownership and stewardship? Committees and councils? Policies and standards? Data quality? Metadata or master data management? Data security and access? Issue management? Technology?
Choose the single element fundamental to fulfill the primary purpose of the program. To do this, answer two questions: what outcomes does the business need and what are the most urgent? From there, codify the primary purpose in your data governance charter and establish its pursuit as the first leg of your roadmap.
Focus on the Purpose and Postpone the Rest
Purpose-driven data governance programs narrow their focus to deliver urgent business needs and defer much of the rest, with a couple caveats. First, data governance programs are doomed to fail without senior executive buy-in and continuous engagement of key stakeholders. Without them, no purpose can be fulfilled. Second, data governance programs must identify and gain commitment from relevant (but perhaps not all) data owners and stewards, but that doesn’t necessarily mean roles and responsibilities need to be fully fleshed out right away.
(ANTSTUDIO/Shutterstock)
Identify the primary purpose then focus on it – sounds like a simple formula, but it’s not obvious. Many data governance leaders are quick to define and pursue their three
practices or five pillars or seven elements, and why shouldn’t they? They need those capabilities, but wanting it all comes at the sacrifice of getting it now. Generate business value with your primary purpose before expanding.
I’ve consulted with multiple customers whose different symptoms trace back to the same blight: a tech company that couldn’t get the trust in trusted data, an insurer that struggled to improve data quality, and a multinational financial services firm that couldn’t remain compliant.
Each approached data governance with a big bang approach instead of simplistically and practically.
Trusted Data
A tech company described their struggles to me this way: in quarterly business reviews cross-functional leaders couldn’t agree on the numbers, what metrics meant, where to source them, or how to interpret them. The primary purpose of their data governance program needed to be to improve trust in the data. Their pathway looked like:
- Identify the critical data elements in dispute
- Negotiate and agree to data definitions; codify them in business glossaries
- Make those data elements available in source of truth datasets (if you show up to QBR with a different number, you’re wrong)
- Repipe widely used dashboards to the source of truth
Simplicity. The data governance elements missing from this pathway were everything that didn’t swiftly move the needle on trusted data. What use are policies without trust? Why improve the quality of data that no one understands?
Data Quality
(GN ILLUSTRATOR/Shutterstock)
An insurer explained to me their dashboards weren’t always refreshed, and when they were, wide fluctuations in values made it impossible to make informed decisions. Their primary purpose needed to be to improve the quality of the data. Their pathway looked like:
- Identify unreliable dashboards and critical data elements
- Uncover the most adversely impacting data quality dimensions, for instance:
- Data Timeliness – Frequently failing pipelines without service-level agreements (SLAs) mean users can’t gauge data staleness
- Data Accuracy – Improper tooling to exchange data over a hybrid multi-cloud environment leads to data interpretation inconsistency
- Benchmark data quality measurements; put profiling and dashboarding in place
- Begin the search for the right hybrid multi-cloud technology vendor
- Improve data quality: define SLAs, reschedule pipelines, standardize data assets
Simplicity. Missing from the pathway were committees and councils, which might improve quality, but not as directly or quickly as attacking the root cause head on.
Regulatory Compliance
For a financial services firm, new regulations were inconsistently interpreted and wastefully implemented across the globe. Their primary purpose needed to be to become truly compliant. Their pathway looked like:
- Establish data governance committees, councils, and working groups with clear responsibilities and accountabilities
- Document policies and standards around compliance definitions and implementation methods
- Implement and enforce
Remember, keep it simple, purposeful, and laser focused, and evolve as business needs change. That way you build your pillars while delivering business value continually.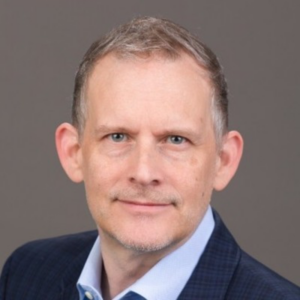
About the author: Shayde Christian is the Chief Data Officer for Cloudera, where he guides data-driven cultural change to generate maximum value from data. He enables Cloudera customers to get the absolute best from their Cloudera products such that they can generate high value use cases for competitive advantage. Previously a principal consultant, Shayde formulated data strategy for Fortune 500 clients and designed, constructed, or turned around failing enterprise information management organizations. Shayde enjoys laughter and is often the cause of it.
-